Thank you for registering.
Webinar
Radionuclide-Sequestration-in-MOFs-DFT-Method
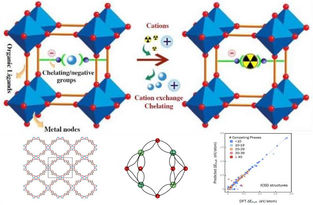
Presented by Shubham Pandey
September 20th*, 21st*, 22nd*
*Live Q&A
Check your email for links and calendar
This webinar will be focused on two key scientific thrusts:
Sequestration of radionuclides in metal-organic frameworks (MOFs) using density functional theory
Predicting the energy and stability of known and hypothetical crystals using a graph neural network
The high porosity, modularity, and structural diversity of MOFs, make them attractive candidates for selective sensing, chemical separation, and radionuclide sequestration. VASP calculations have been used to determine the energetic favorability of Tc, U, Th, Am, and Cm ion exchanges at Zr-, U-, and Th- sites in MOF clusters. A convenient procedure can be employed using builders for subdividing MOFs into their secondary building units (SBUs) and organic linkers. A range of DFT methods within MedeA VASP, including the most efficacious exchange-correlation functionals, DFT+U corrections, vdW interactions, and relativistic and magnetic effects can then be employed to establish the reliability of the results. Various reference states of ions including vacuum, continuum water media, and explicit water molecules surrounding the ions have also been explored. Substitution of Tc, Am, Cm, and Th with MOF constituent ions is found to be energetically favorable in all the MOF structures that were examined, using explicit water molecules surrounding the ions as a reference state. The electronic structures of MOF-SBUs along with the origins of the band gaps in MOFs (SBU or linker) have also been investigated using their partial densities of states.
A key point in this work is that the discovery of new inorganic materials in unexplored chemical spaces necessitates calculating total energies quickly and with sufficient accuracy to assess relative stabilities. Machine learning models that provide such a capability for both ground-state (GS) and higher-energy structures have proven instrumental in accelerating the screening of new materials over vast chemical configuration spaces. Here, the importance of a balanced training dataset of GS and higher-energy structures is demonstrated, accurately predicting the total energies of new materials using a generic graph neural network architecture. ~16,500 density functional theory (DFT) total energies from the NREL Materials Database were combined with ~11,000 DFT energies of hypothetical structures generated in-house were used in training the model, ensuring that the training set is not biased towards either GS or higher-energy structures. The resulting GNN model satisfactorily ranks different polymorphic structures for a given composition in the correct order of their energies. This work illustrates the utility and power of DFT and Machine Learning in discovering and understanding new materials and additional opportunities and applications will be discussed.